The Rise of AI in Various Industries
Artificial Intelligence (AI) is defined as the ability of machines or computer systems to perform tasks that typically require human intelligence. In recent years, AI has been increasingly utilized across various industries, such as healthcare, finance, transportation, and retail.
The use of AI technology has led to both positive and negative impacts on society. On the one hand, it has allowed for improved efficiency and accuracy in decision-making processes.
On the other hand, it raises ethical concerns related to bias, accountability, and transparency.
Diverse sectors are taking advantage of the potential benefits that AI offers.
In healthcare, for example, AI-powered systems can help doctors diagnose illnesses more accurately and efficiently than human doctors alone.
The financial sector uses machine learning algorithms to detect fraudulent activities in real-time while transportation companies can deploy autonomous vehicles that can reduce traffic congestion while increasing safety on the roads.
Why Discussing the Ethics of AI is Important
Despite the numerous benefits that come with utilizing AI technology across different industries, there are also important ethical considerations that must be taken into account. Ethical concerns surrounding bias in algorithmic decision-making processes have come to light due to several high-profile cases where algorithms have been found to exhibit racial or gender biases. It is important for stakeholders involved in developing or deploying these technologies to be aware of these ethical considerations.
Additionally, discussions surrounding accountability have emerged as a result of incidents involving self-driving cars causing accidents due to software glitches or errors made by drivers who rely too much on autonomous systems without fully understanding their limitations.
There is also a need for transparency when it comes to how these technologies work, so people can make informed decisions about whether they want them integrated into their lives.
An Overview of Main Ethical Concerns: Bias, Accountability & Transparency
There are three main ethical concerns surrounding the use of AI:
- bias,
- accountability,
- and transparency.
Bias refers to the tendency of machine learning algorithms to make decisions that reflect or reinforce existing societal biases.
Accountability relates to who is responsible for the actions of AI systems and ensuring that they are held accountable when things go wrong.
Transparency refers to how much information we have about the decision-making processes that underlie AI systems.
Bias, accountability, and transparency should be considered together when discussing ethical issues related to AI as they are often interconnected. An algorithm may not be transparent if it has been trained on biased data, which means it may also have accountability issues if it harms certain groups in society unfairly due to their race, gender or any other characteristic.
Overall, as technology continues to advance and shape our lives more profoundly than ever before, there is an urgent need for discussions around ethical considerations related to AI technology.
The following sections will delve into each of these three main ethical concerns in more detail so that you can gain a better understanding of each issue’s complexities.
Bias in AI
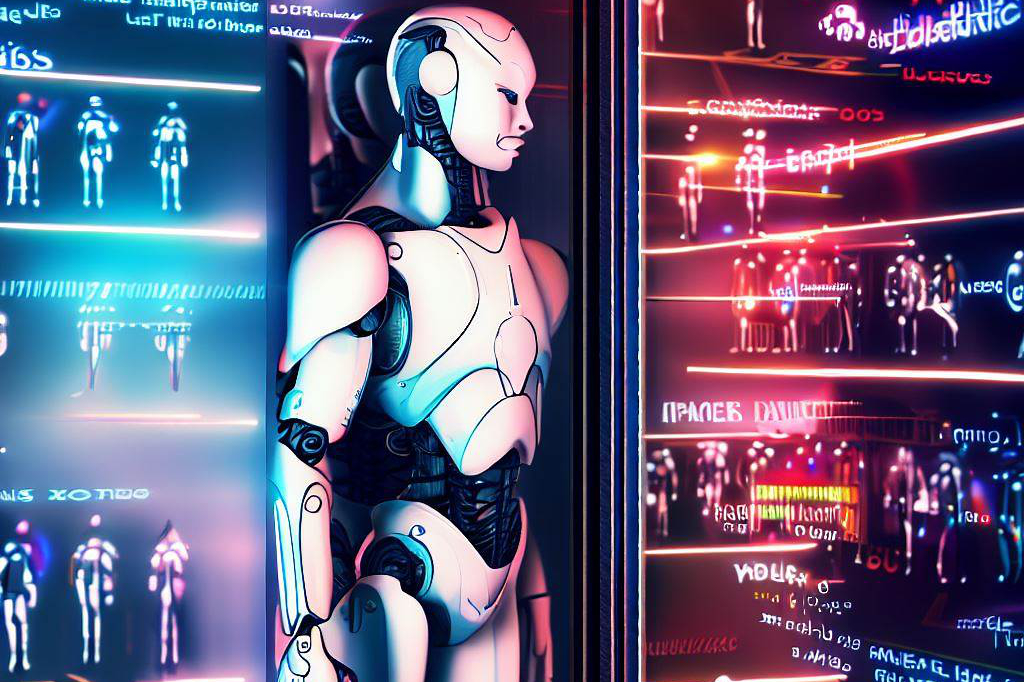
What is Bias in AI?
Bias in AI refers to the tendency of artificial intelligence systems to reproduce or amplify existing societal inequities, often without the explicit intention of doing so. These biases can be introduced into AI systems through a variety of means, including biased training data or assumptions made by the developers.
For example, if an AI algorithm is trained on a dataset that is predominantly male, it may have difficulty accurately recognizing female faces or voices.
Similarly, if an algorithm used in hiring decisions is trained on data that reflects historical hiring biases (such as favoring candidates who attended certain universities), it may perpetuate those same biases.
Examples of Biased AI Systems and Their Impact
There are numerous examples of biased AI systems with potential negative impacts on society.
One notable example involved a predictive policing algorithm used by the New York Police Department that was found to disproportionately target Black and Hispanic individuals due to the biased data used for training. This led to increased scrutiny and ultimately resulted in the program’s termination.
Another example can be seen in facial recognition technology, which has been found to have higher error rates for individuals with darker skin tones and women. This has significant implications for law enforcement and surveillance technologies that rely on facial recognition as a tool.
The use of biased algorithms can perpetuate discrimination against historically marginalized groups and further entrench societal injustices. It is important for developers and policymakers alike to recognize these risks and work towards minimizing bias within AI systems.
Mitigating Bias in AI Systems
There are several strategies for mitigating bias within AI systems, including:
1. Diversifying datasets: By ensuring that datasets are representative of diverse populations, developers can help mitigate the risk of biased algorithms.
2. Auditing algorithms: Regularly auditing algorithms for unintended consequences or discriminatory outcomes can help identify and address biases.
3. Building in transparency: By making AI systems more transparent, users can better understand how they function and identify biases or errors.
Overall, it is crucial for developers to recognize that AI systems are not neutral and can perpetuate societal biases if not developed and deployed with care. Strategies such as diversifying datasets, auditing algorithms, and building in transparency can help mitigate these risks.
Accountability in AI
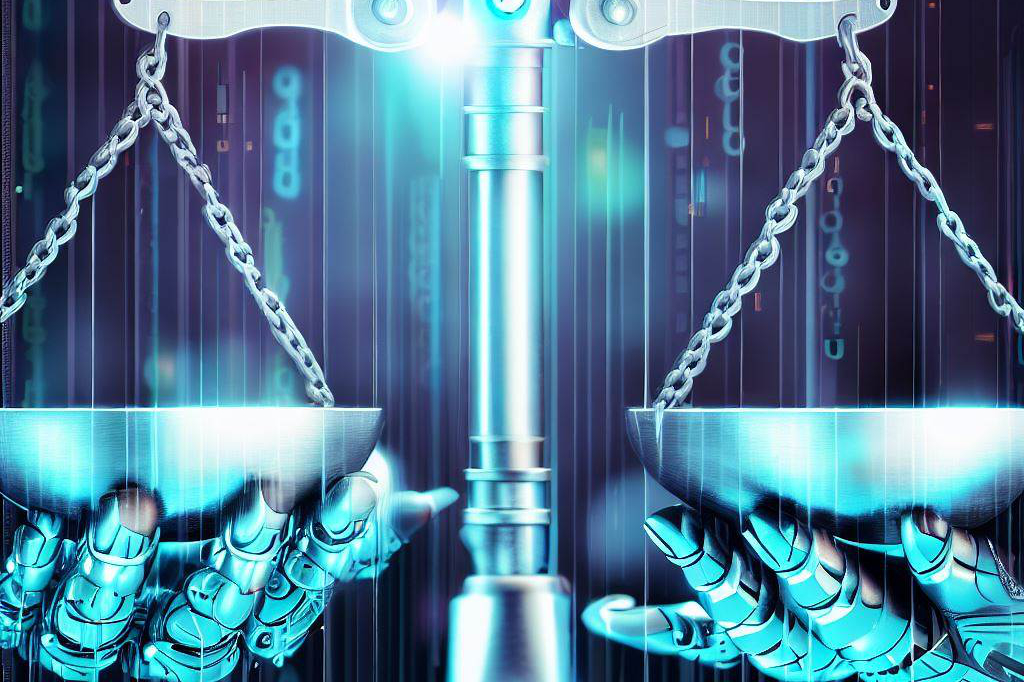
Artificial intelligence has the potential to transform almost every aspect of our lives, from healthcare and education to finance and transportation. However, with this great power comes great responsibility. One of the main ethical concerns surrounding AI is accountability; that is, who should be held responsible for the actions of a machine learning system or an algorithm that causes harm or makes a mistake?
Definition of accountability and why it is important for AI systems
In general, accountability refers to the obligation of individuals or organizations to take responsibility for their actions and decisions. In the context of AI, accountability means determining who should be held responsible if an AI system causes harm or makes a mistake.
This can include developers who created or trained the system, those who deployed it in a particular domain, or those who use it in practice. The importance of accountability in AI cannot be overstated.
As these systems become increasingly autonomous and complex, they can make decisions that have significant consequences for individuals and society as a whole. For example, an algorithm used by police departments to predict crime rates may lead to biased targeting of certain racial groups if not properly audited for fairness.
Challenges in holding individuals or organizations accountable for the actions of an AI system
One major challenge in holding individuals or organizations accountable for the actions of an AI system is determining exactly where responsibility lies.
With traditional software development, it may be more straightforward to assign blame since individual lines of code can often be traced back to specific developers. However, with machine learning algorithms that can learn from vast amounts of data on their own and make decisions autonomously based on that data, pinpointing blame becomes much more difficult.
Additionally, current legal frameworks are often ill-equipped to handle cases where an AI system causes harm. Many laws rely on human agency as a prerequisite for being held liable; however, some AI systems are designed to operate with minimal human intervention, making it unclear who should be held accountable if something goes wrong.
Proposed solutions for ensuring accountability in the development and deployment of AI systems
To ensure accountability in the development and deployment of AI systems, several proposed solutions have been put forth. One idea is to create clear guidelines and standards for ethical AI development and deployment that companies must follow. This can include processes for transparency, testing for bias, and auditing algorithms on a regular basis.
Another proposed solution is to develop legal frameworks that hold individuals or organizations accountable for the actions of their AI systems. This can include updating current laws or creating new ones specifically tailored to the unique challenges presented by autonomous systems.
Some experts have suggested creating mechanisms for oversight and regulation of AI systems that involve collaboration between government agencies, industry leaders, and other stakeholders. This could involve creating an independent organization responsible for auditing AI systems to ensure they are being developed and deployed ethically.
Ensuring accountability in the development and deployment of AI systems is critical to minimizing the potential harm they may cause. While there are many challenges in holding individuals or organizations accountable for the actions of these complex algorithms, several proposed solutions offer hope that we can create a future where artificial intelligence is both powerful and ethical.
Transparency in AI
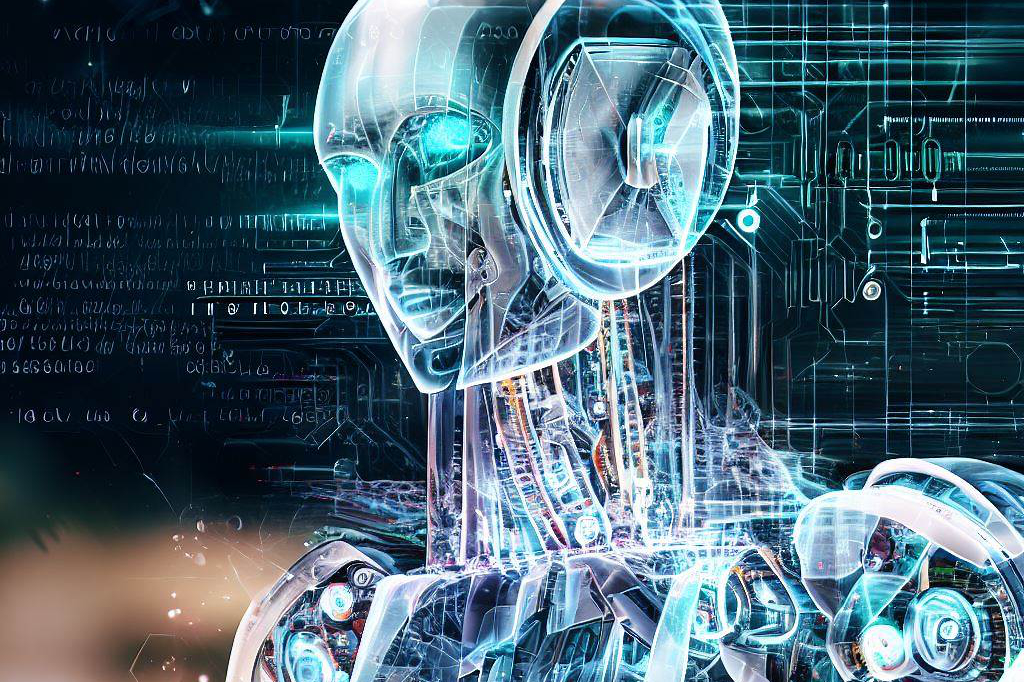
Artificial intelligence has become an increasingly popular technology in recent years. It is used in various industries to automate processes and make predictions based on data analysis.
Transparency is an essential ethical concern that comes into play when considering the development and use of AI systems. In this section, we will explore the definition of transparency, why it is important for users to understand how an AI system works, the challenges in achieving transparency, and proposed solutions for increasing it.
Why Transparency is Important
Transparency refers to the ability of users to understand how a decision was made by an AI system.
This understanding includes knowledge of the data used, algorithms applied, as well as any relevant variables or factors considered during decision-making.
Transparency helps promote fairness by giving users insights into why decisions were made and whether they were biased or influenced by factors that may be undesirable.
Transparency also helps build trust between users and developers of AI systems since they can see how decisions are made and if they align with their goals or values.
Moreover, transparency allows individuals affected by decisions made by AI systems to hold those responsible accountable for their actions.
Challenges in Achieving Transparency
One major challenge in achieving transparency with complex algorithms and machine learning models is their inherent complexity. The ability of these models to process vast amounts of data at high speed means that their internal workings can be very difficult to understand, even for trained professionals.
Another challenge arises when dealing with sensitive data such as personal medical records or financial information. There are often strict privacy concerns around this type of data, which can limit its availability for review purposes, affecting one’s ability to obtain a complete picture of what happened within an algorithmic system.
Proposed Solutions for Increasing Transparency
One proposed solution for increasing transparency is developing explainable AI systems that are designed from the ground up with transparency in mind. Explainable AI systems are built with transparency and interpretability in mind, allowing users to understand the decision-making process and the reasoning behind it.
Another approach is to promote open access to data and algorithms used in AI systems.
This will enable independent audits of these systems, leading to increased accountability, and fairness.
There is a need for better documentation of the data used in developing AI systems.
This includes a clear description of how the data was collected, its quality, as well as any relevant biases that may have been introduced into the system through its use. Comprehensive documentation can increase transparency by allowing individuals to understand how decisions were made when using an AI system.
Final Thoughts
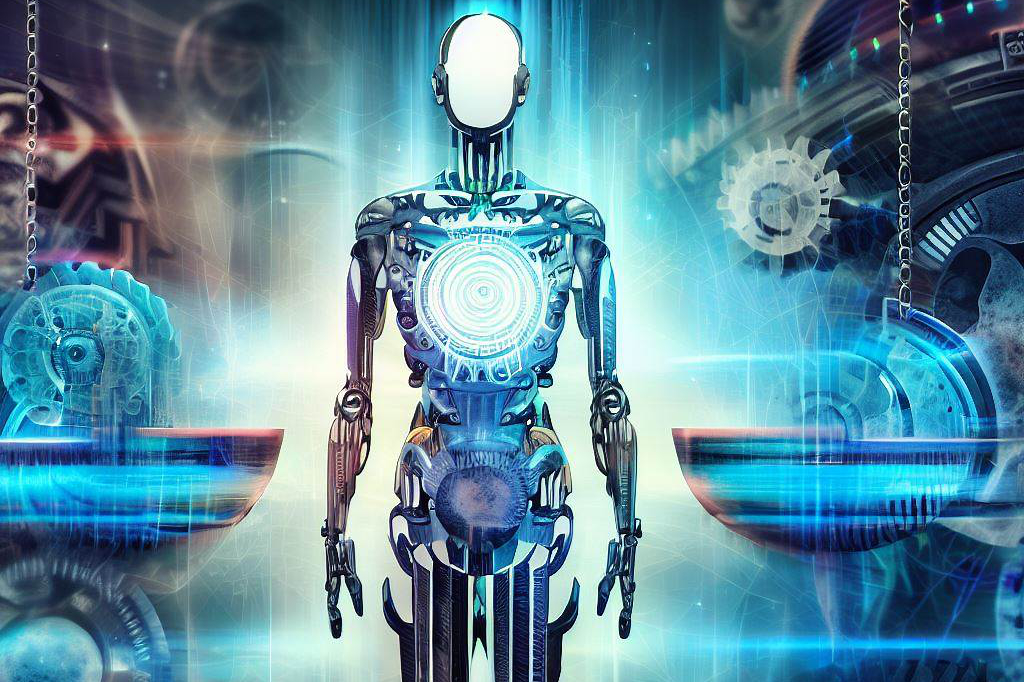
As AI becomes more prevalent in our daily lives and industries, it is crucial that we continue to have discussions about its ethical implications. This includes addressing issues such as bias in data training sets, accountability for the actions of an AI system, and transparent communication about how decisions are made.
The development of new policies and regulations may also be necessary to ensure that the deployment of AI systems aligns with ethical standards. Looking forward, there is reason to be optimistic about the future of AI ethics.
Increased awareness and discussion around these issues can lead to positive changes in the industry. By engaging in ongoing conversations about bias, accountability, and transparency in AI systems, we can work towards creating a world where technology benefits all members of society fairly.
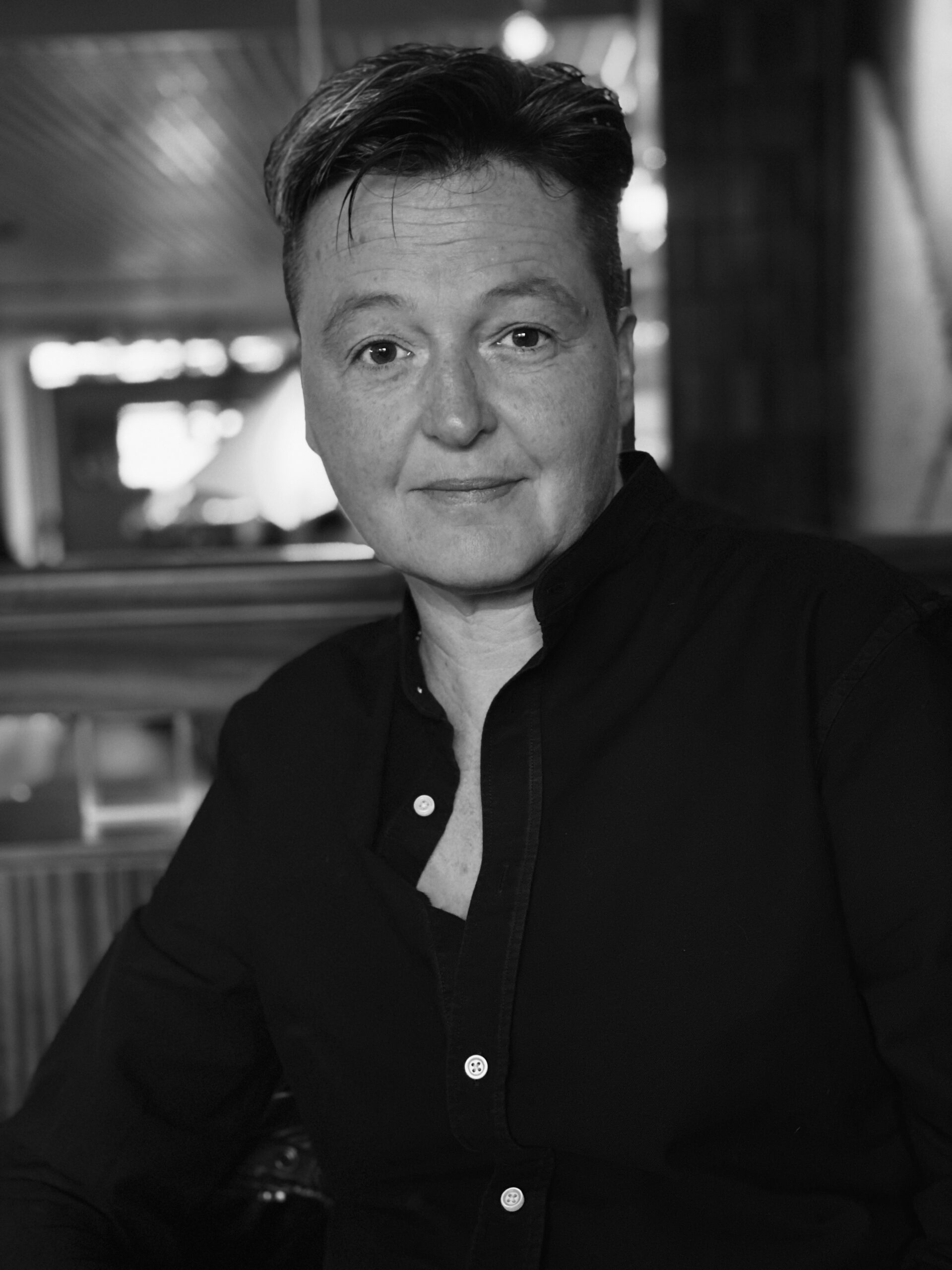
C M, a seasoned editor, journalist, and consultant, is deeply fascinated by the convergence of technology, space, and the future of humanity.
With a particular interest in transhumanism, futurology, and the philosophical and ethical dimensions of these domains, C M serves as the lead contributor to TranscendSphere and SpaceSpotlight.
When not penning insightful articles on these rapidly evolving fields, C M indulges in their love for podcasts and books, proudly embracing their status as a ‘Happy Nerd Extraordinaire!’