Prosthetics have been in use for centuries, with documented evidence dating back to ancient Egypt. In recent years, prosthetic technology has advanced significantly, with improvements in materials, design, and functionality.
However, despite these advancements, prosthetics still have limitations that can impact the wearer’s quality of life. Limitations include difficulties with adaptability and ease-of-use.
Amputees may struggle to adjust to their new prosthetic limb and experience discomfort or pain during use. Additionally, controlling the prosthetic limb and performing tasks can be challenging.
An amputee’s ability to perform daily tasks impacts their independence and quality of life. Machine learning is an area of artificial intelligence that involves developing algorithms that enable computers to learn from data without being explicitly programmed.
Machine learning has applications in many fields, including healthcare. In the context of prosthetics, machine learning has the potential to improve adaptability and ease-of-use by enabling machines to learn from data collected from amputees’ interactions with their prosthetic devices.
Prosthetics and Their Limitations
Prosthetics are devices designed to replace a missing body part or function. They can be external or internal devices used for a variety of purposes, such as
- mobility aid (e.g., walking),
- cosmetic (e.g., breast prosthesis),
- or functional replacement (e.g., hand prosthesis).
While they have come a long way since ancient times when they were made out of wood or metal, modern-day prosthetics still have limitations that can affect users’ quality of life. One limitation is adaptability; it takes time for amputees to get used to using their new limbs fully.
Even after adjustments are made for comfort and fit, individuals may still experience phantom pain sensations or have trouble using them naturally in place of the lost appendage. Additionally, there are challenges with fine motor control and overall coordination, which can make daily tasks challenging.
Ease-of-use is another limitation. Some prosthetics are complicated to control or require a lot of physical effort to move.
The process of fitting and adjusting prosthetics can be lengthy and expensive, requiring multiple appointments with healthcare professionals. All these barriers can discourage some amputees from using prosthetic devices altogether.
Brief Overview of Machine Learning
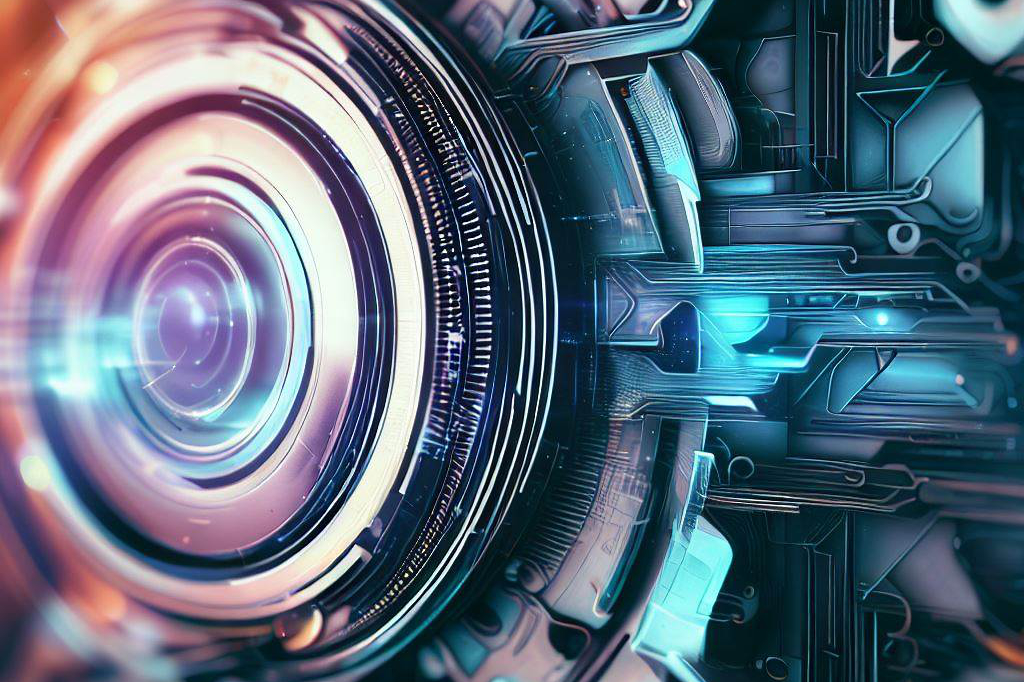
Machine learning is an area of artificial intelligence that involves the development of algorithms that allow computers to learn from data. These algorithms can detect patterns in data and use them to make predictions or decisions without being explicitly programmed.
Machine learning has applications in many fields, including healthcare. In healthcare, machine learning can help with tasks such as identifying diseases in medical images or predicting patient outcomes based on clinical data.
In the context of prosthetics, machine learning has the potential to revolutionize the field by enabling machines to learn from amputees’ interactions with their devices. This technology could help address limitations related to adaptability and ease-of-use by improving how prosthetic limbs respond to users’ movements and making them more intuitive for amputees to control.
Machine Learning in Prosthetics
Definition and Explanation of Machine Learning
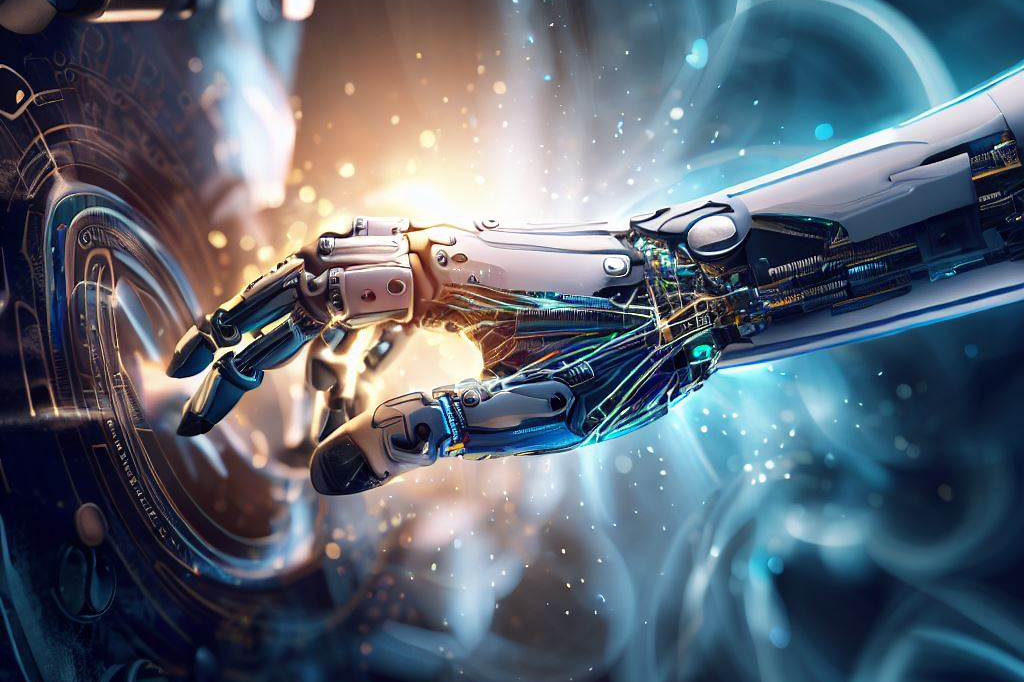
Machine learning is a branch of artificial intelligence that involves the development of algorithms and statistical models that enable machines to automatically learn from data inputs without being explicitly programmed. Machine learning algorithms use statistical techniques to identify patterns and relationships in large sets of data, enabling machines to make informed decisions based on this data. In the context of prosthetics, machine learning can be used to analyze data from sensors embedded within prosthetic limbs, such as accelerometers and gyroscopes.
By analyzing this data, machine learning algorithms can learn how users interact with their prosthetics and make predictions about what movements they are likely to make next. This predictive capability allows for more precise control over the prosthetic limb.
How Machine Learning Can Be Applied to Prosthetics
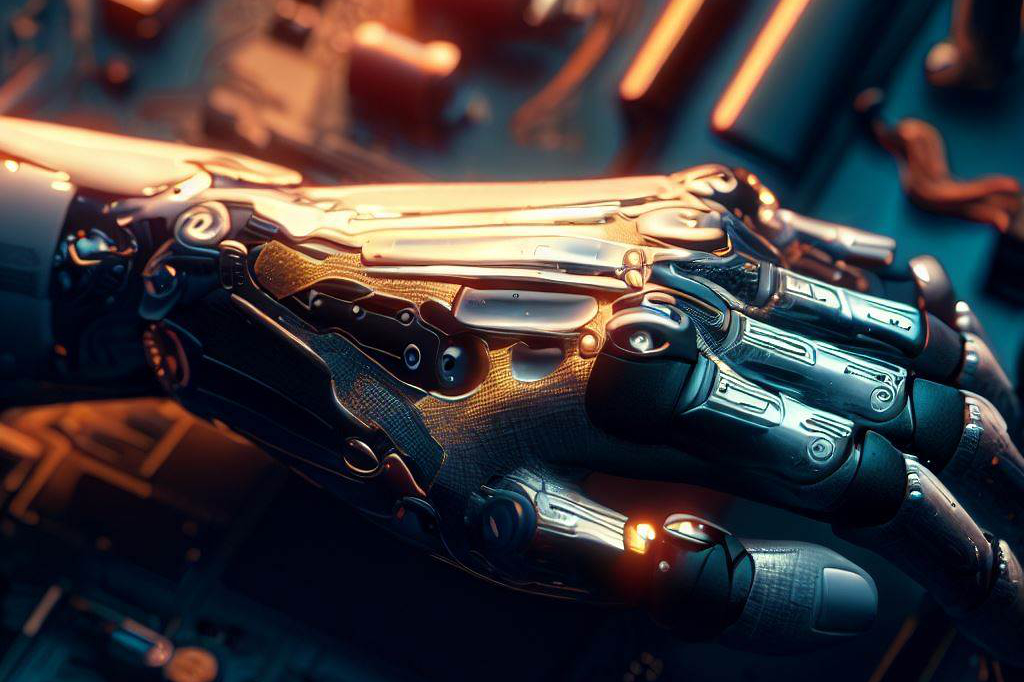
Machine learning can be applied in a variety of ways in the field of prosthetics. One application is in pattern recognition – by analyzing sensor data from a prosthetic limb, machine learning algorithms can generate predictions about what movement the user intends to make based on patterns detected in previous movement data. This allows for more fluid control over the limb.
Another application is personalized adaptation – by analyzing sensor data over time, machine learning algorithms can detect changes in user behavior and adapt the functionality of the prosthetic accordingly. For example, if a user starts using their prosthetic limb more frequently for certain tasks, the algorithm may adjust its settings to better accommodate those tasks.
Machine learning can be used to predict wear-and-tear on a prosthetic limb. By analyzing usage patterns over time, machine learning algorithms can predict when certain components may need replacement or repair before they fail completely.
Benefits of Using Machine Learning in Prosthetics
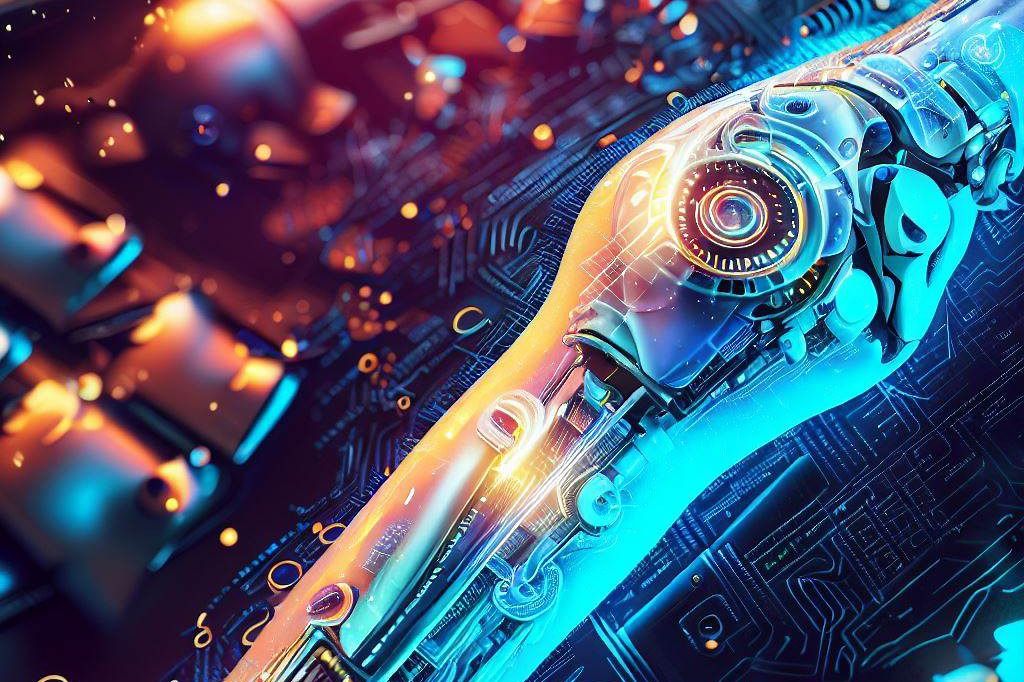
The benefits of using machine learning in prosthetics are numerous. Firstly, it allows for greater adaptability and personalization – users can have a more tailored experience with their prosthetic limb, improving their overall quality of life. Secondly, it enables greater precision and control over the limb, allowing for more complex movements and greater functional versatility.
It can help reduce the need for frequent adjustments or repairs by predicting wear-and-tear before it becomes a major problem. Machine learning has the potential to significantly enhance the functionality and ease-of-use of prosthetic limbs.
Improving Prosthetic Adaptability with Machine Learning
Challenges faced by amputees when using prosthetics
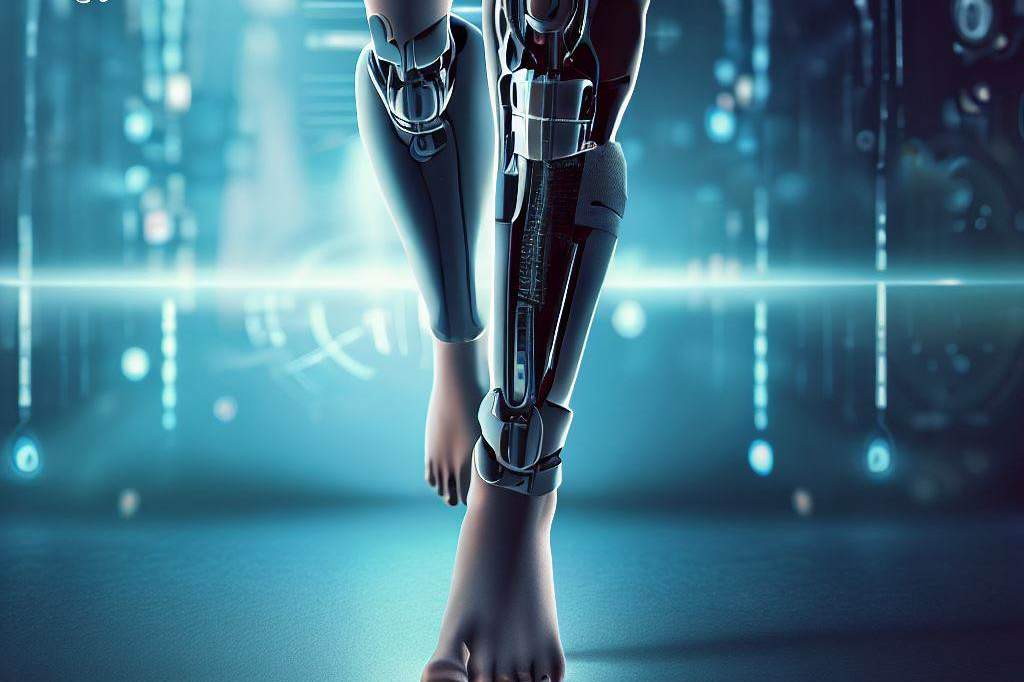
Amputees face several challenges when using prosthetics, such as limited mobility, difficulty adjusting to the device, and a lack of natural movement. One of the main issues is that prosthetic devices are often not adaptable to an individual’s needs and preferences.
This can lead to discomfort, frustration, and decreased use of the device. Another challenge is that prosthetic devices require significant physical effort from the user.
The user has to constantly adjust their body movements to accommodate the limitations of the device. This can result in fatigue and reduced quality of life for amputees.
How machine learning can help improve adaptability
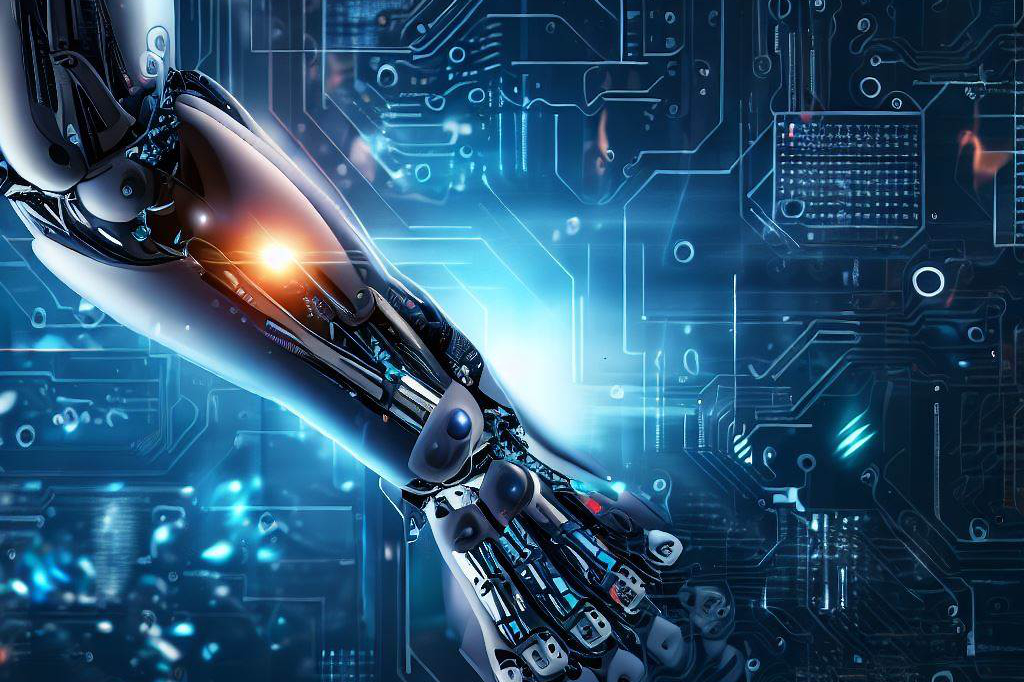
Machine learning algorithms can be used to analyze data from sensors embedded in prosthetic devices, such as accelerometers and gyroscopes. By analyzing this data, machine learning algorithms can identify patterns in how an individual moves with their device.
This information can then be used to customize the device for each individual user. For example, a machine learning algorithm could identify that an individual tends to move their arm in a specific way when they reach for an object.
The algorithm could then adjust the prosthetic arm’s movement so that it matches this pattern more closely. This would make it easier for the user to control the device and perform daily tasks more efficiently.
Examples of how machine learning has been used to improve adaptability
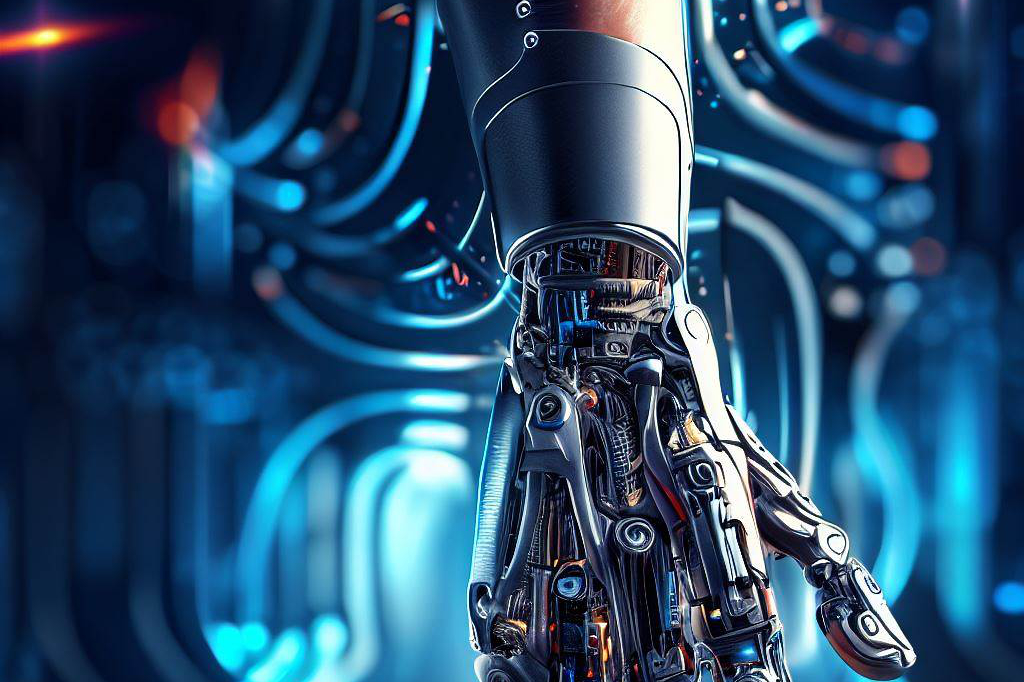
Researchers have already made significant progress in using machine learning algorithms to improve prosthetic adaptability.
For instance, a study conducted by researchers at the Georgia Institute of Technology found that a machine learning algorithm was able to adjust a powered ankle prosthesis based on real-time measurements of its wearer’s walking gait. This resulted in improved walking performance compared with traditional ankle prosthesis designs.
Another example is the use of machine learning to develop an adaptive prosthetic hand. Researchers at Case Western Reserve University used a machine learning algorithm to analyze data from sensors embedded in a prosthetic hand.
The algorithm was able to predict how the user intended to move their fingers and adjust the prosthesis accordingly. This resulted in improved functional performance for users, such as being able to grasp and hold objects with greater ease.
Overall, the use of machine learning to improve prosthetic adaptability has great potential for revolutionizing the field of prosthetics. By enabling devices to adapt to each individual’s needs and preferences, amputees can experience greater comfort, increased mobility, and a higher quality of life.
Improving Ease-of-Use with Machine Learning
Common Issues Faced by Amputees When Using Prosthetics
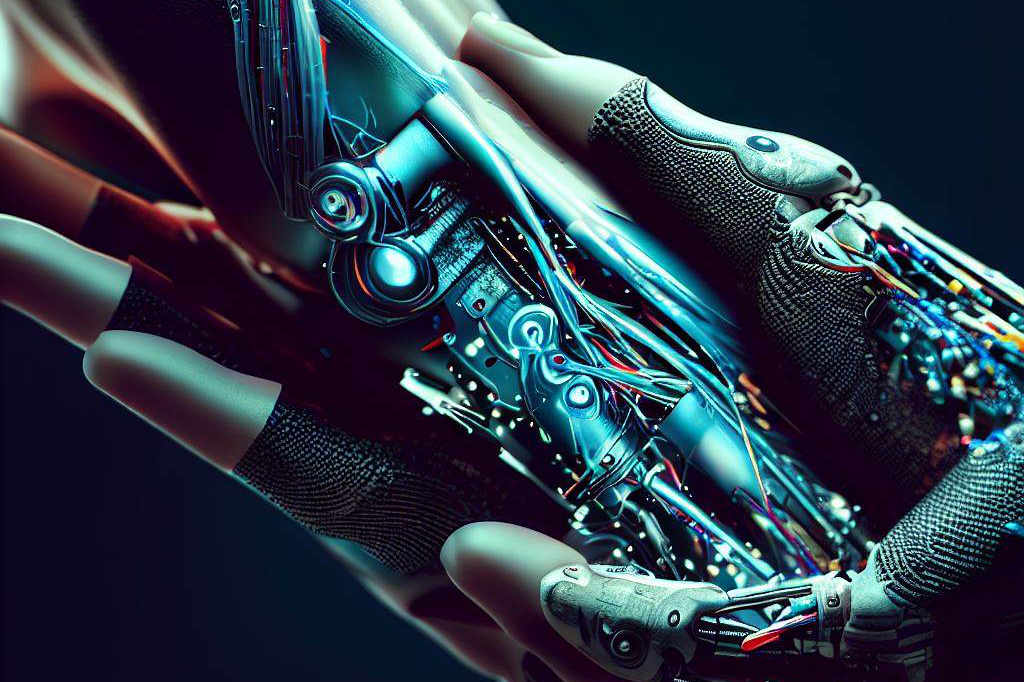
Despite the advancements in prosthetic technology, many amputees still face significant challenges when using their prosthetics. One of the most common issues is discomfort caused by poor fit or improper alignment of the prosthetic device. This can lead to skin irritation, pressure sores, and even infection.
Additionally, some amputees struggle with identifying the correct movement patterns needed to control their prosthetic device. This can result in frustration and difficulty using the device effectively.
Another issue arises when an amputee tries to use a new prosthetic or one that is more advanced than what they are used to. It can take time for them to adjust to the new features and controls, which can be frustrating and time-consuming.
How Machine Learning Can Help Improve Ease-of-Use
Machine learning algorithms can help improve ease-of-use for amputees by analyzing data from sensors on the devices they are using and adjusting settings accordingly. For example, machine learning algorithms can analyze data on how an amputee moves their remaining limb along with data from sensors on the prosthetic device to adjust alignment or fit in real-time.
Additionally, machine learning algorithms can help identify patterns in amputee’s movements that may indicate ineffective use of their devices or improper fit. Based on these patterns, suggestions for adjustments could be recommended for optimal use.
Examples of How Machine Learning Has Been Used to Improve Ease-of-Use
One example of how machine learning has been used to improve ease-of-use is through a study conducted at MIT, where researchers used machine learning algorithms to analyze data collected from sensors embedded within a robotic prosthesis worn by a patient over several months. The algorithm was able to identify patterns in the patient’s movements that reflected an over-reliance on compensatory movements from their non-amputated limb.
The researchers then used the data to develop targeted exercises to help the patient improve their movement patterns and better utilize their prosthetic device.
Another example comes from a company called Coapt, which uses machine learning algorithms to predict user intent and adjust prosthetic control accordingly.
The algorithms analyze muscle activity in the residual limb to determine what specific movement the user intends to make. This allows for more intuitive control of the prosthetic device and allows amputees to perform complex tasks more easily.
Overall, machine learning has great potential for improving ease-of-use for prosthetic users. As research and development in this field continue, we can expect to see even more advancements that will help amputees live fuller lives with greater independence.
Future Developments in Machine Learning and Prosthetics
Current research on the topic
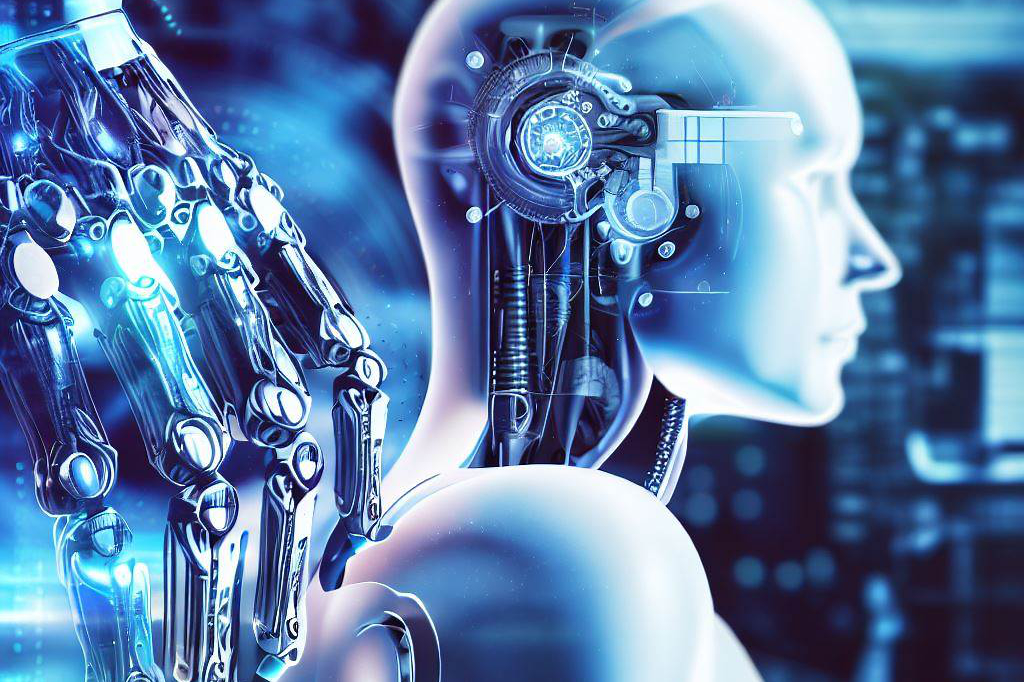
There is currently a lot of ongoing research into the use of machine learning in prosthetics. Some of the most promising developments include the use of sensors and other technologies to gather data that can be used to improve adaptability and ease-of-use. For example, researchers are exploring ways to use machine learning algorithms to analyze data from sensors on prosthetic limbs, allowing them to better understand how amputees move their limbs and adapt to different situations.
Another area of research involves using machine learning algorithms to predict how an individual amputee will perform with a particular prosthetic device. This could involve analyzing factors such as their age, weight, level of physical fitness, and other variables that could impact their ability to use a prosthetic limb effectively.
Potential advancements that could be made through further development
With continued research and development, there is enormous potential for machine learning to revolutionize the field of prosthetics. One area where significant advancements could be made involves creating prosthetic devices that are more intuitive and user-friendly. This might involve developing new interfaces or control systems that allow amputees greater control over their devices or incorporating more advanced sensors or other technologies into the devices.
Another potential area for advancement is improving the durability and reliability of prostheses. Machine learning algorithms could help identify patterns in usage data that indicate when a device is likely to fail or require maintenance, allowing users or healthcare providers to take action before any issues arise.
It’s clear that machine learning has tremendous potential when it comes to improving prosthetic adaptability and ease-of-use. With continued investment in research and development, we can expect even greater advances in this field in years to come.
Although there are certainly challenges still facing researchers working in this area, including issues around data privacy and ethical considerations, the potential benefits to amputees are simply too great to ignore. By working together to overcome these challenges, researchers and healthcare professionals can help improve the lives of countless individuals around the world who rely on prosthetic devices every day.
Final Thoughts
The impact of future developments in machine learning on the field of prosthetics is significant. As research continues, advancements will likely lead to even more sophisticated systems that can better understand a user’s intentions and movements through sensors that are integrated with artificial intelligence algorithms. This could lead to more natural movements for amputees using prosthetic devices and an overall improvement in quality of life for those affected by limb loss.
Additionally, further development in this area could reduce healthcare costs related to follow-up appointments and maintenance due to fewer breakdowns or malfunctions. The potential benefits are vast, from improved functionality for individual users, increased accessibility for those who might not have had access before due to cost or geography barriers, as well as improved psychological health through increasing self-confidence with abilities previously lost due to limb loss.
Importance of Continued Research and Development in This Area
There is a clear need for continued research and development in this area. While the potential benefits are vast, there are still limitations to current technology, and further advancements in machine learning could help overcome these limitations.
Additionally, continued research will help ensure the safety and efficacy of prosthetic devices that incorporate machine-learning technology. Furthermore, improving the ease-of-use and adaptability of prosthetic devices can have a significant impact on the quality of life for those affected by limb loss.
By reducing barriers to mobility and increasing independence, amputees can return to activities they enjoyed pre-amputation. As such, continued research into machine learning and prosthetics should be a priority area for researchers working towards enhancing health outcomes for people with disabilities.
While there is much work to be done in this field, incorporating machine learning into prosthetic devices shows great promise in improving the functionality of these devices for amputees. The potential impact on quality of life is vast, and researchers should continue exploring this avenue as a means of improving healthcare outcomes for those affected by limb loss.
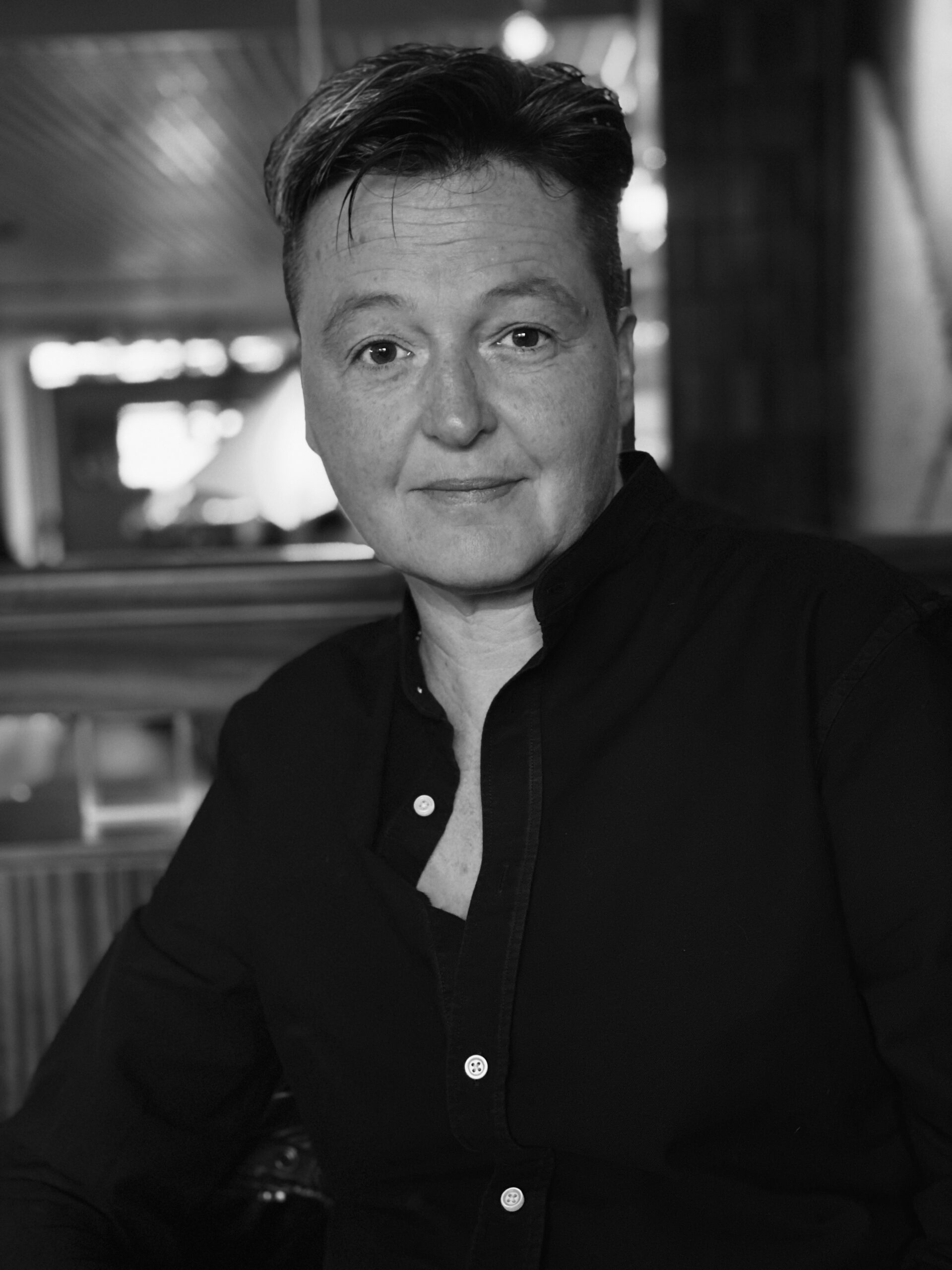
C M, a seasoned editor, journalist, and consultant, is deeply fascinated by the convergence of technology, space, and the future of humanity.
With a particular interest in transhumanism, futurology, and the philosophical and ethical dimensions of these domains, C M serves as the lead contributor to TranscendSphere and SpaceSpotlight.
When not penning insightful articles on these rapidly evolving fields, C M indulges in their love for podcasts and books, proudly embracing their status as a ‘Happy Nerd Extraordinaire!’